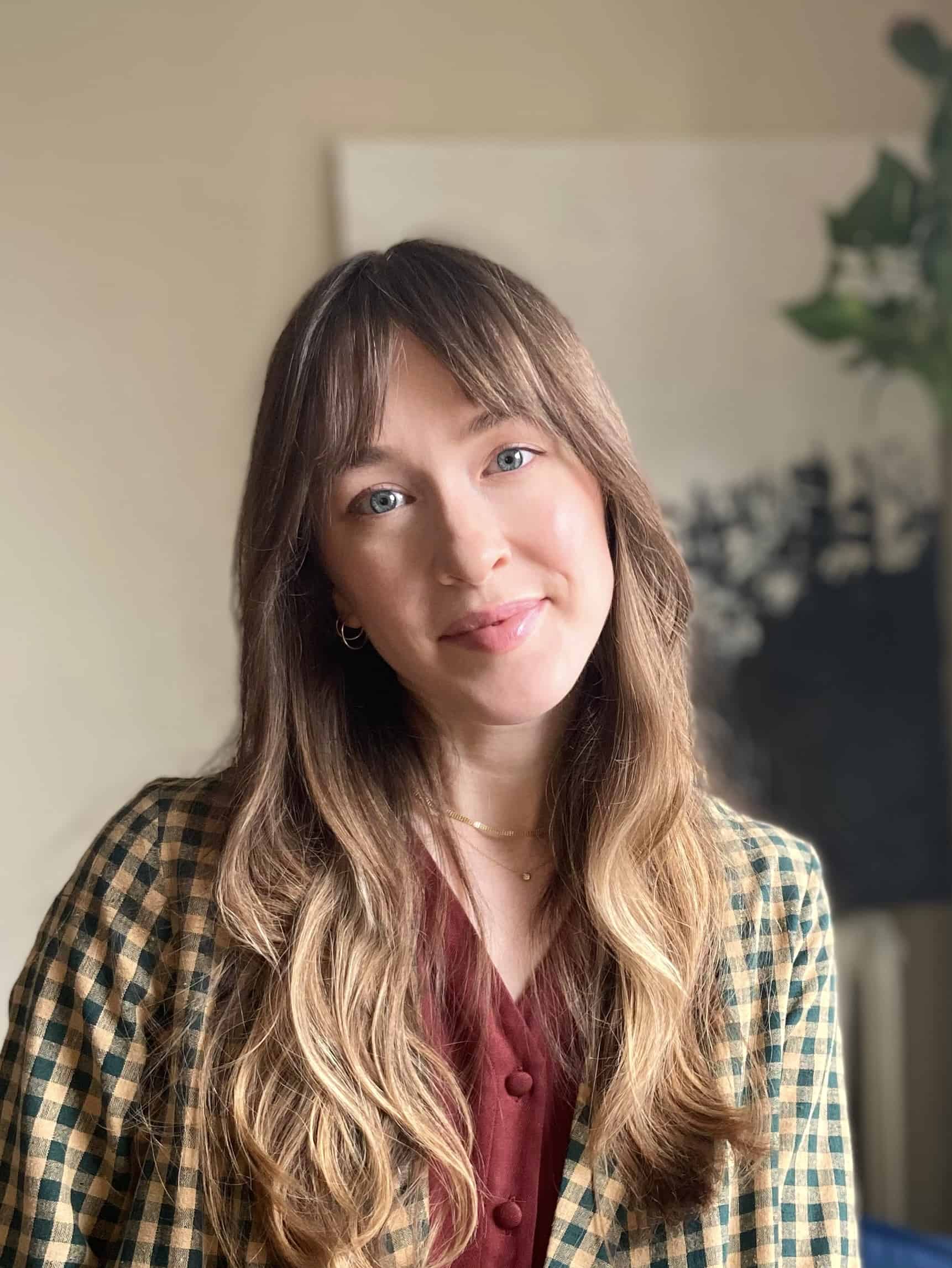
Hannah Hilligoss is a student at Harvard Law School and a member of the Labor and Employment Lab.
It’s no secret that the hiring process is rife with bias and discrimination. Hiring personnel are only human, after all, and their personal biases lead to both unintentional and intentional discrimination. But what if hiring personnel weren’t human? What if we could replace them with objective, data-driven algorithmic assessments? No more bias! Or at least that’s what some vendors of this technology claim. Ironically, the only way this claim could be true is if the very problem vendors are trying to solve for—human bias—didn’t exist. This is because, as Cathy O’Neil puts it, “data processes codify the past. They do not invent the future.” In technical terms, algorithmic hiring systems rely on machine learning: techniques that “detect patterns in existing data (called training data) to build models that forecast future outcomes in the form of different kinds of scores and rankings.” If the system “learns” from past hiring decisions that exhibit some degree of bias, the system’s forecasted outcomes will exhibit the same pattern of human bias. For example, one notorious algorithmic resume screening tool found that the two factors most predictive of employee success were (1) your name was Jared, and (2) you played lacrosse. In other words, the algorithm was selecting for affluent, white men, reflecting the skewed pool of past employees.
Algorithmic hiring tools are affecting everyone from Hilton hospitality and service workers to Goldman Sachs investment bankers. Given the pervasiveness of these tools, it is concerning that a recent survey of prominent algorithmic hiring system vendors found that only 38% explicitly discussed the potential for bias and bias mitigation measures. This is disturbing, according to one author of the report, because it means the vendors are either “not thinking about [bias] at all, or they’re not being transparent about their practices.” If vendors are not proactively mitigating algorithmic discrimination, then they are actively reproducing and exacerbating systemic discrimination in the hiring process.
These systems are automating every step of the hiring process (sourcing, screening, and interviewing). If we want to prevent the automation of hiring practices that have resulted in the hyper-masculine and racist culture of corporate America, we must intervene in the rollout of algorithmic hiring tools.
SOURCING
Developing an applicant pool is the first step in the hiring process, and human resources departments are increasingly relying on AI-powered job boards and advertising platforms—like LinkedIn, ZipRecruiter, and Facebook—to source applicants. This trend is likely to accelerate as employers seek to save time as they hire from a more remote, less geographically constrained, and, therefore, larger pool of applicants in the wake of COVID-19.
While techniques vary across platforms, most allow employers to set initial parameters that target and exclude people with certain attributes (years of experience, graduate degree, skills, etc.) from seeing their job posting. The platforms then offer “audience expansion” or “lookalike audience” features that use data provided by users or inferred from their online activities to show job advertisements to users similar to the employer’s target audience. Finally, platforms use machine learning to predict which users are most likely to interact with the job posting.
With active intervention in defining target parameters, these tools could be used to expand and diversify job applicant pools. Without active intervention, these tools will replicate demographic employment patterns within homogenous sectors like tech, domestic work, and construction. For example, Dolese Bros. Co., construction company in rural Oklahoma, used Facebook’s (new and improved) special ads portal to advertise job openings. Of the 20,000 people who saw the ad, 87% were men. From a machine learning standpoint, this makes sense: the model predicted which Facebook users were likely to apply for the Dolese construction job based on the traits of current construction workers. And over 95% of current construction workers are male. While it’s unclear how many women would have responded to the job ad, “not informing people of a job opportunity is a highly effective barrier” to employment.
SCREENING
Next, hiring personnel “screen” applicants to determine who to interview. This is the “most active area of development” for algorithmic hiring system vendors. Many algorithmic screening tools analyze resumes, but some use gamified skills assessments to cull or flag certain applicants based on their performance. Resume screening algorithms are often trained on data consisting of resumes of current and past employees paired with their job performance metrics (like sales numbers or rate of promotion). Amazon, for example, scrapped an algorithmic resume screening tool because, after being trained on 10 years of past Amazon applicant resumes, it determined that men were preferable to women. Specifically, resumes of applicants who went to all-women’s colleges or who were involved in “women’s” clubs or sports were penalized. Again, this is unsurprising given that top tech companies like Amazon, Facebook, Apple, Google, and Microsoft were anywhere from 60 – 80% male at the time. For all the algorithm know, these companies disproportionately hired men in the past because men were better, not because of personal or systemic discrimination against women.
INTERVIEWING
After screening, a job applicant will likely be interviewed. Many companies now conduct video interviews—either with or without a human interviewer involved—through tools like HireVue, which leverage facial recognition technology and natural language processing to rate and recommend applicants. These tools “statistically link” interview data—facial movements, word choice, and voice tone—with competencies related to emotional intelligence, cognitive ability, and general personality. The models are trained on and target variables are defined by data from a company’s current employees. Enough companies are using HireVue that some colleges now instruct students on “how to impress” the algorithm.
It is well documented that facial recognition technology is less accurate for Black women than for white men: one prominent study found error rates of up to 34.7% for darker-skinned women, while the maximum error rate for light-skinned men was 0.8%. Even if the HireVue’s psychometric assessments accurately predicted employee success—which is contested and is the subject of an FTC complaint—it is likely that they would be less accurate for some applicants than others, resulting in racial and gender discrimination.
IS ALL HOPE LOST?
Human hiring personnel are biased. As are algorithmic hiring tools. So where do we go from here? Technochauvinists—those who believe that technology is always the solution—may remain hopeful that algorithms can be completely “de-biased.” This is unlikely for both societal and technical reasons. More likely, according to Ruha Benjamin, is that relying on algorithms to solve fundamentally human problems will usher in the New Jim Code: technical solutions that “hide, speed up, and even deepen discrimination, while appearing to be neutral or benevolent when compared to the racism of a previous era.” While this doesn’t mean we should give up on developing new hiring processes that root out bias and discrimination, it does mean we should be pump the brakes on implementing technical solutions. At least until we can be sure that they work as intended without perpetuating discrimination, and until we (or Congress) can require meaningful transparency and accountability from vendors and some amount of recourse for those harmed along the way.
Daily News & Commentary
Start your day with our roundup of the latest labor developments. See all
March 31
Trump signs executive order; Appeals court rules on NLRB firing; Farmworker activist detained by ICE.
March 28
In today’s news and commentary, Wyoming bans non-compete agreements, rideshare drivers demonstrate to recoup stolen wages, and Hollywood trade group names a new president. Starting July 1, employers will no longer be able to force Wyoming employees to sign non-compete agreements. A bill banning the practice passed the Wyoming legislature this past session, with legislators […]
March 27
Florida legislature proposes deregulation of child labor laws, Trump administration cuts international programs that target child labor and human trafficking, and California Federal judge reversed course and ruled that unions representing federal employees can sue the Trump administration over mass firings.
March 25
Illinois warehouse quota bill vetoed; Minnesota residents organize; circuit split on NLRB deference continues
March 23
Mahmoud Khalil and labor; CA Fast Food Council's slow start; debating worker-to-worker organizing
March 19
Colorado unions push to join Montana on just cause protection, Starbucks advocates for the Counterman standard